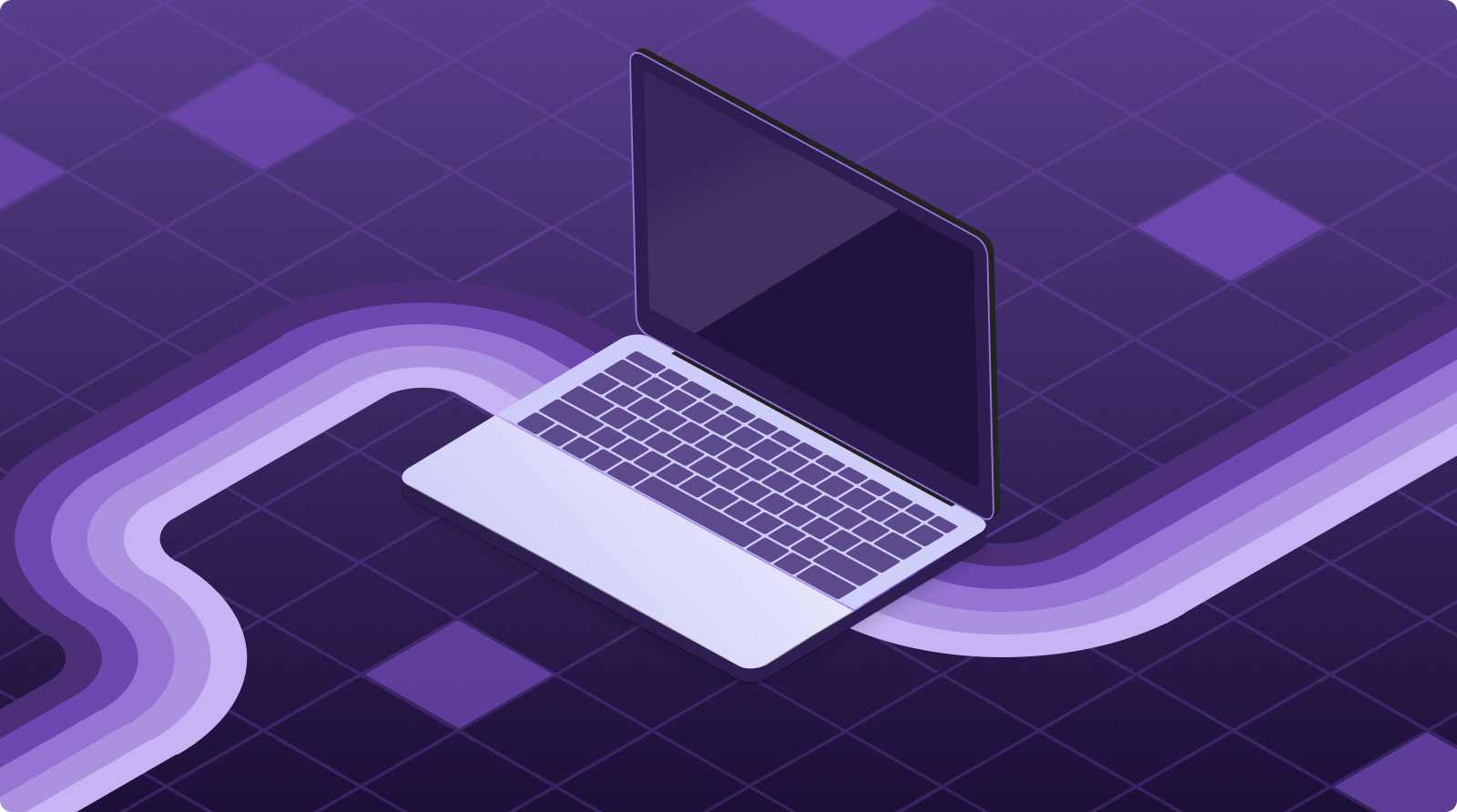
This webinar introduces the Astro SDK, an open source tool for rapid and clean pipeline development that allows you to seamlessly transfer and transform data without system-specific or Airflow-specific code.
The Astro SDK has undergone tons of development, one major release, and many minor releases since this event. For a more up to date overview of the project, check out our Simplified DAG Authoring with the Astro SDK webinar or this getting started tutorial.
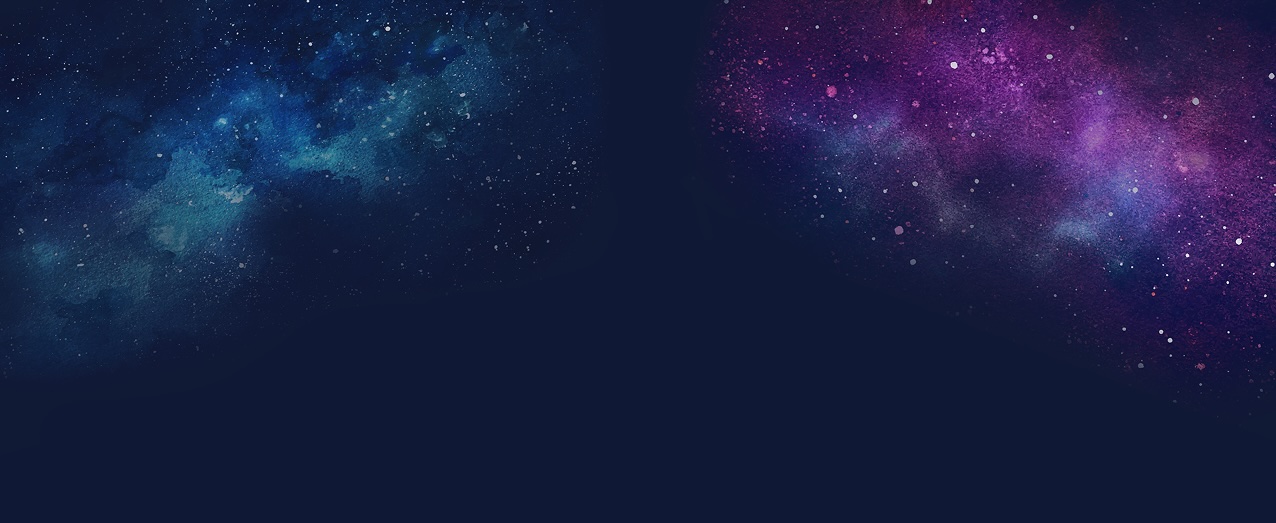