Laurel’s Timekeeping Transformation with AI and Airflow
Laurel partnered with Astro to provide their data team with more time to focus on building pipelines, accelerating their ML pipeline development.
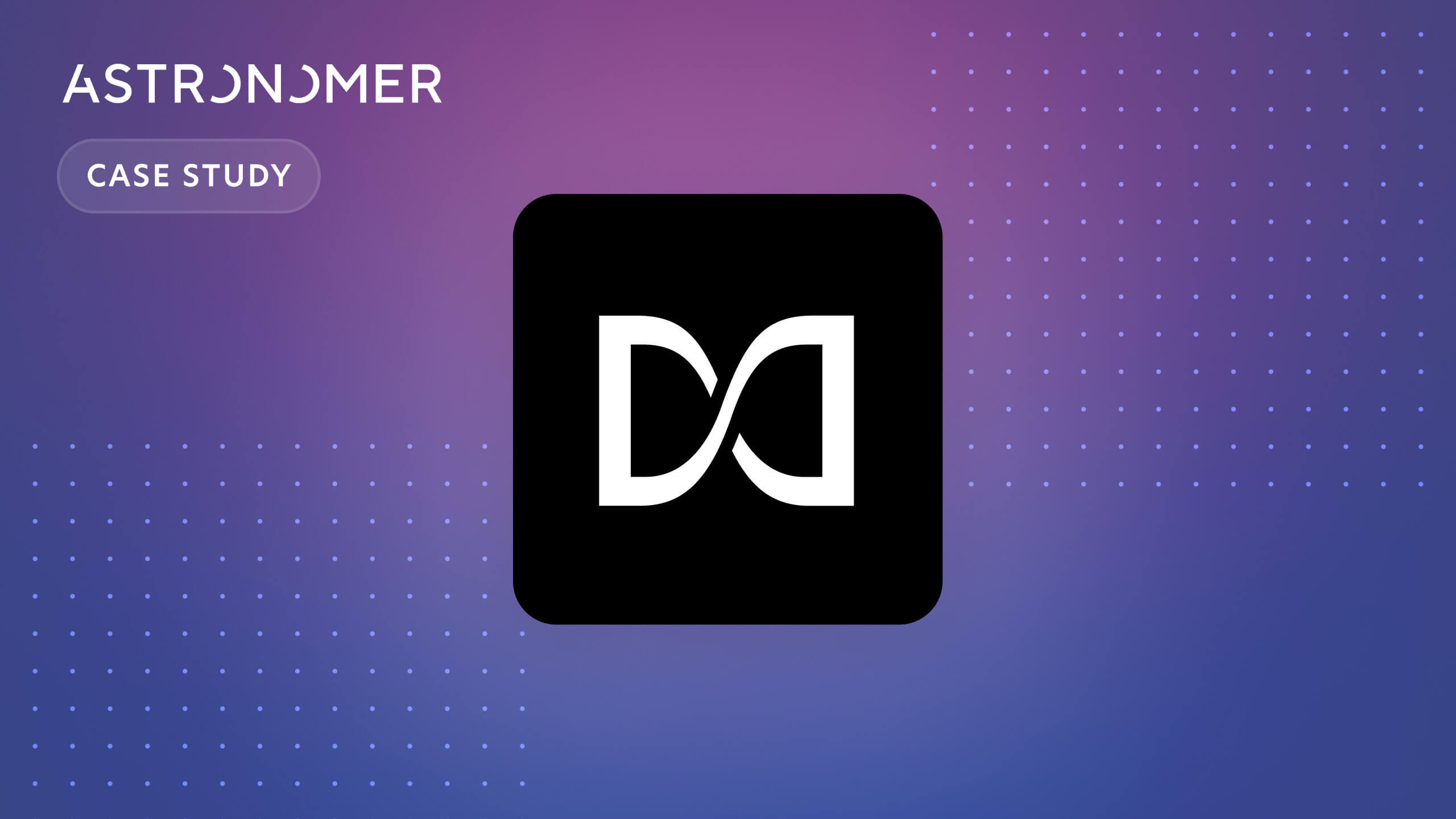
The Challenge: An Industry Trust Deficit in Hourly Billing
One of the most pressing issues in hourly billing is the eroded trust between service providers and purchasers. On one hand, service purchasers often harbor doubts about the diligence of providers, leading to a desire to audit their work. Conversely, service providers feel the expectations constantly shift, necessitating appropriate compensation.
This mistrust, especially pronounced in high billable rate areas like legal services, has led to intricate challenges. For instance, lawyers bill in six-minute increments to maintain credibility about how their time is spent, turning timekeeping into a cumbersome task. Enter Laurel, a pioneering company dedicated to building trust in timekeeping. As Andy Ward, VP, Product and Data at Laurel, pointed out,”It turns out nobody likes keeping their time. They recognize that it’s a necessary evil but it’s a task that people hate.”
At its core, Laurel aims to tackle the timekeeping challenges faced by professionals worldwide. Their initial focus was on lawyers for two primary reasons:
- Among all professionals who bill their time hourly, lawyers tend to have the highest hourly billable rate. Thus, any additional time found in their day translates to a substantial bottom-line impact.
- The company’s founder, being a lawyer himself, had an intimate understanding of the challenges and nuances of the profession.
As Laurel ventured into sectors like accounting and consulting, they discovered even more resonance with their product. Laurel introduced a system that meticulously constructs a “digital footprint” of a professional’s daily activities. By seamlessly integrating with tools like Zoom, Microsoft Teams, and Exchange, Laurel captures the essence of what a professional did on their computer during the day. Laurel can also help their customers use this gathered workflow data to evaluate their operations and drive further profitability, making it a win-win.
The underlying principle is both simple and revolutionary. As Ward put it,“Why should you tell your computer what work you did on your computer, when your computer can tell you?” This approach not only ensures pinpoint accuracy in timekeeping but also directly addresses the primary reasons many professionals, especially lawyers, detest traditional timekeeping methods.
The Solution: Embracing Airflow and Astronomer
According to Ward, Laurel’s decision to harness Airflow and collaborate with Astronomer stemmed from a need for a catalyst in their processes.
The initial workloads they embarked on were primarily ETL (extract, transform, load) workloads and data lake creation tasks to clean and store time keeping data for analysis. They would then take this data, and use it to train Machine Learning (ML) models to predict what people were doing with their time based on the actions being performed on the computer. The amount of data gathered to power these models for each user is massive. Having a robust and scalable system to manage these pipelines was paramount for their ML team, who were previously bogged down by manual data extraction and ETL tasks.
“Since many of the data engineers on the team had worked with Airflow in the past, it was quickly selected as the tool of choice, as it had the scalability, flexibility, and reliability to handle enterprise-scale workloads. We also decided to go with a managed Airflow solution instead of self-hosting to give the data team maximum time back to build their pipelines,” explained Ward.
After exploring a few different solutions and not finding them satisfactory, they were referred to Astronomer by one of their other vendors. Their team then dove head first into a trial with Astro, leveraging their existing Airflow expertise and Astro’s easy-to-use functionality to create a minimum viable product (MVP) of ML model retraining pipeline.
Rapid and reliable technical support was vital for Laurel. “When choosing to purchase a solution rather than building it in-house, we expect a better service level than what we could provide internally,” Ward asserted.
During their trial, the Laurel team was able to build a complete ML model retraining pipeline from scratch on Astro, with any questions or problems promptly answered by the Astronomer support team. This confirmed to them that Astronomer was the right platform to live at the center of their modern data stack.
After adopting Astro, the Laurel data team now had a powerful tool to act as the center of their modern data stack, eliminating manual workloads and enabling them to start building new pipelines to better serve their business. As they began building these new pipelines and onboarded more and more customers, Astro’s auto-scaling capabilities made sure that their Airflow environments were able to handle the increased load efficiently without compromising on performance.
“The surface area that we’re touching is quite large, so Airflow acting like a single pane of glass for our data to put all this together has been really helpful for us,” summarized Ward.
Airflow as the Center of the Modern Data Stack for Machine Learning Operations (MLOps)
Instead of using monolithic large language models for ML predictions, Laurel takes the approach of having many smaller ML models for individual customers and use cases, with ML driving every aspect of Laurel’s product. By employing a multitude of models, they can tailor each one to address distinct tasks.
This modular approach not only allows for greater flexibility and precision but also facilitates quicker updates and iterations. If one model needs adjustments or encounters issues, it can be refined without disrupting the entire system. “I firmly believe that small, hyper-specific models and ensemble approaches are considerably superior to generalist models for any business application,” emphasized Ward.
Furthermore, as Laurel serves different users, firms, and verticals, having specialized models ensures that each segment receives a solution optimized for its unique requirements, enhancing the overall user experience and efficiency.
One example of these models is Laurel’s “tap to complete” model, which constructs a dictionary based on narratives written by timekeepers. This model helps provide suggestions that match the writing style of the user. Another model checks narratives constructed by lawyers for compliance, ensuring they align with narratives that get paid. This reduces write-offs and improves billing efficiency. Before implementing this model, firms would have multiple full-time employees handling this task, but with the model, the process is not only automated but also literally twice as efficient.
As you can imagine, having this many distinct models across both customers and use cases can introduce a ton of complexity if not managed properly. That’s where Airflow comes in, sitting at the core of Laurel’s ML platform, it manages all steps of the model lifecycle, from data acquisition to model training, validation and deployment.
Ward explained, “The automation provided by Airflow allows for frequent model updates, which can feel almost magical to users as they observe their changes to the models take effect almost immediately.”
“We use Airflow as the central component of our MLOps to orchestrate every aspect of our ML pipelines, from automated model testing and experimentation to deployment, evaluation, and promotion,” Ward further elaborated.
Airflow’s automation and speed has helped the team to meet both external and internal KPIs. Externally, the main KPI is “time to time,” which measures how long it takes to create a timesheet, which Airflow was immediately able to impact by automating all of the previously manually executed data pipelines. Internally, the team’s focus was on development velocity, which is measured by how quickly they can test a model’s hypothesis. By enabling more frequent model updates, and thus allowing the team to test new hypotheses faster, Airflow was able to greatly improve this metric as well. On top of that, Airflow’s extensibility gives the Laurel team the flexibility to orchestrate the best AI tools for their models, without being pigeon-holed into a particular solution.
Finally, Astro’s ability to grow and scale along with Laurel’s customer base has been crucial in supporting their business growth, as each new customer means more pipelines and data to manage. Astro makes that process both efficient and performant, which is key for Laurel’s business proposition. As Andy Ward succinctly put it, “Thanks to Airflow, we can onboard more customers, more features, faster!”
Looking Forward
As Laurel continues to grow, they aim to further automate processes. The end goal is for users to no longer need to look at their time cards because the system would have generated them automatically. This would require numerous ML models, and rather than hiring more data scientists, the plan is to orchestrate these clear checks using tools like Airflow.
Laurel’s journey with Airflow and Astronomer is a testament to the transformative power of the right tools in amplifying business processes. By addressing the age-old problem of timekeeping with cutting-edge solutions, Laurel has not only elevated its own operations but has also delivered immense value to professionals spanning various sectors.
The Company
Laurel is a pioneering artificial intelligence (AI) company that automates time and billing for professional services. The company's platform employs AI to automatically create timesheets for time spent on specific matters by capturing and analyzing clean data. This enables users and organizations to understand where time is being spent and to provide more accurate and structured time data.
The Challenge
Laurel's Machine Learning team grappled with manual data extraction, ETL tasks, and the need for efficient management of workloads, including data lake creation and cleaning, for timekeeping data analysis and ML model training.
The Astro Solution
Laurel chose the managed Airflow solution over self-hosting to provide their data team with more time to focus on building pipelines. The combination of Astro's user-friendly functionality, Astronomer's Airflow expertise, and responsive technical support solidified the decision.
Results
-
Reduced "Time to Time"
Automation of previously manual data pipelines significantly reduced the time required to create timesheets.
-
Improved Development Velocity
With Airflow enabling more frequent model updates, Laurel experienced an accelerated testing pace for model hypotheses.
-
Eliminated Manual Workloads
The adoption of Astro eliminated manual workloads, allowing Laurel to initiate the development of new pipelines to better serve their business.
-
Efficient Auto-Scaling
As new pipelines were built and more customers were onboarded, Astro's auto-scaling capabilities ensured that Laurel's Airflow environments efficiently handled increased workloads without compromising performance.