Introducing Data Quality in Astro Observe: The Orchestration-First Approach to Reliable Data
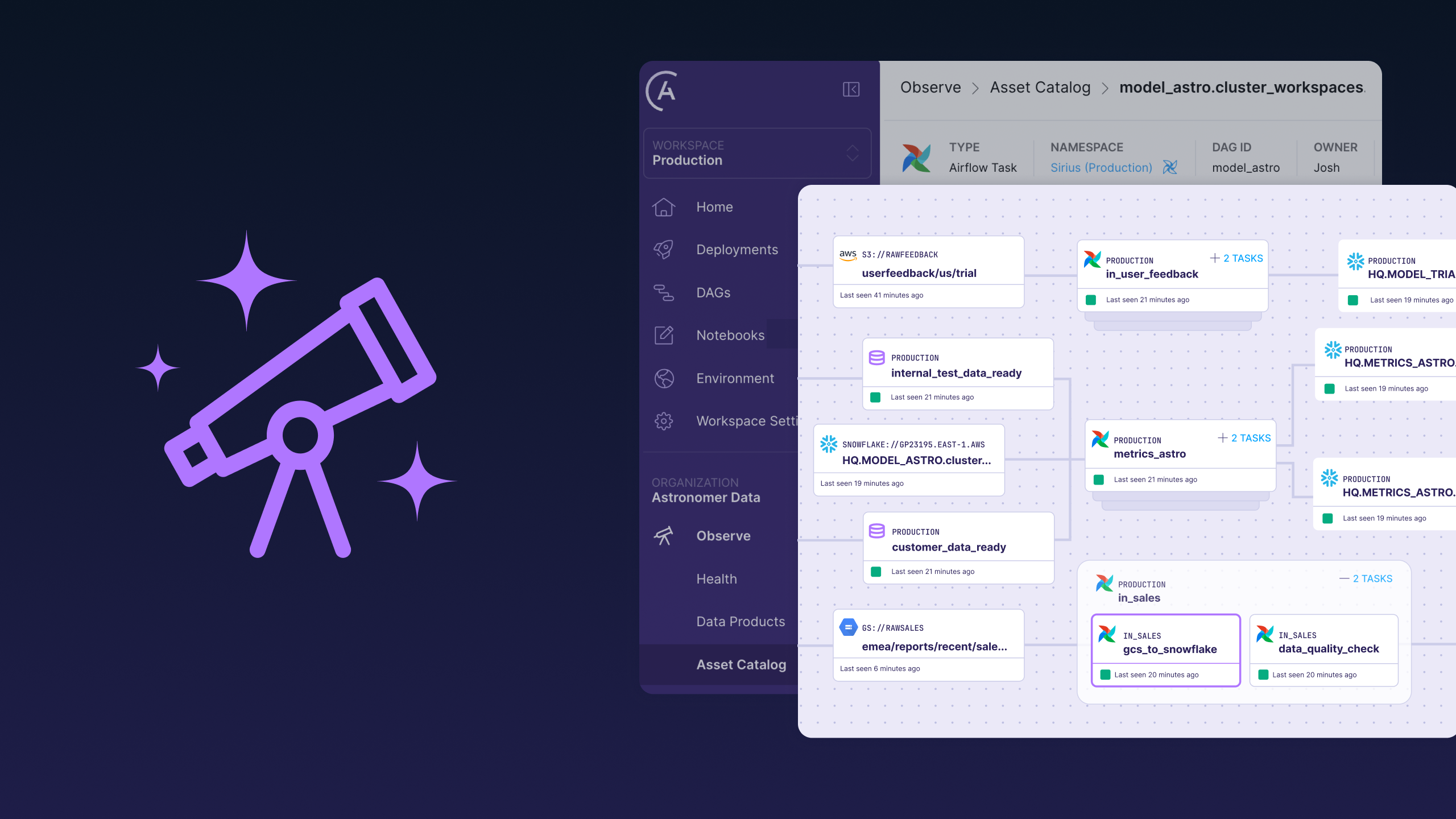
As organizations increasingly rely on data to drive critical business decisions and power AI applications, data quality is no longer just a technical issue – it’s a key business priority. Poor data quality silently erodes business performance across every industry. From delayed decisions and missed opportunities to damaged customer trust and regulatory exposure, the ripple effects touch every corner of your organization.
The Rising Importance of Data Quality
The data landscape has undergone a fundamental shift in recent years. What was once a straightforward flow of information from source systems to reports has evolved into complex ecosystems of pipelines, transformations, and interdependent data products. In this environment, traditional approaches to data quality, isolated checks performed after data lands in warehouses, are increasingly insufficient.
The truth is, data quality cannot be an afterthought. It must be woven into the fabric of how data moves through your organization. This realization has driven many teams to explore various approaches to quality management, from custom frameworks to specialized tools. However, each path comes with its own set of challenges.
The Limitations of Current Approaches
When organizations build custom data quality solutions, they divert engineering resources from business priorities to framework maintenance while creating inconsistent standards across teams. These homegrown approaches struggle with enterprise-scale data volumes, create dangerous knowledge dependencies on specific team members, and require constant updates as data systems evolve.
Standalone data quality tools present their own set of challenges. Most critically, they create disconnected context by monitoring data in isolation from orchestration, which inevitably creates blind spots in the data lifecycle and at times overlapping alerts – forcing teams to toggle between fragmented tools just to piece together the full picture.
Both approaches ultimately result in slower resolution times, incomplete coverage, and a fragmented understanding of your data health. Without direct integration into your orchestration layer, these solutions miss the critical context of how and when your data was processed – leaving teams scrambling to understand where in your data pipeline the problem originated. Ultimately costing precious time and resources while downstream systems continue operating with compromised data.
Announcing Enhanced Data Quality in Astro Observe: From Pipeline Execution to Data Reliability
At Astronomer, we believe the future of data quality lies in an orchestration-first approach. That’s why we’re excited to announce enhanced data quality monitoring in Astro Observe, now available in private preview.
We first introduced Astro Observe as a new way for Airflow users to gain operational and data-level visibility across their pipelines. With this release, we’re expanding that foundation to include built-in data quality monitoring deeply integrated with orchestration.
Unlike traditional tools that monitor data downstream and lack an understanding of how or when that data was produced, Astro Observe embeds quality checks directly into your orchestration platform. This delivers unparalleled contextual awareness, letting you trace data quality issues to specific pipelines, understand root causes, and see the downstream impact of failures in one unified experience.
By having data quality monitors directly within Astro Observe, users can go beyond passive alerting to actively ask and answer critical questions about their data. Want to know which Airflow task caused a schema drift in your Snowflake revenue table? Curious which pipelines are generating the most data quality alerts this week? Need to see all failed checks on high-priority tables used in dashboards? Astro Observe makes it easy to pinpoint failed checks on high-priority tables, monitor row count anomalies, and surface the downstream impact of data issues, all within one integrated view.
Key Capabilities in Private Preview
In this initial release, we’re starting with support for data quality checks in Snowflake, the industry’s leading cloud data warehouse, and will expand to additional platforms in the coming months, including Databricks, BigQuery, and Redshift. Key capabilities include:
- Comprehensive Data Quality Checks: Set monitors to track data volume (row volume), data completeness (column null percentages), and schema drift with configurable thresholds, schedules, and alert severity to understand the criticality of failures.
- Proactive, Contextual Alerting: Get alerted automatically when Data Quality checks or thresholds are breached for proactive troubleshooting with the full pipeline execution context needed to speed resolution.
- Unified Asset Catalog with Quality Metrics: Automatically view data warehouse tables alongside Airflow tasks and datasets with table popularity scores that highlight usage patterns – helping teams prioritize data quality checks on the most critical and frequently accessed assets.
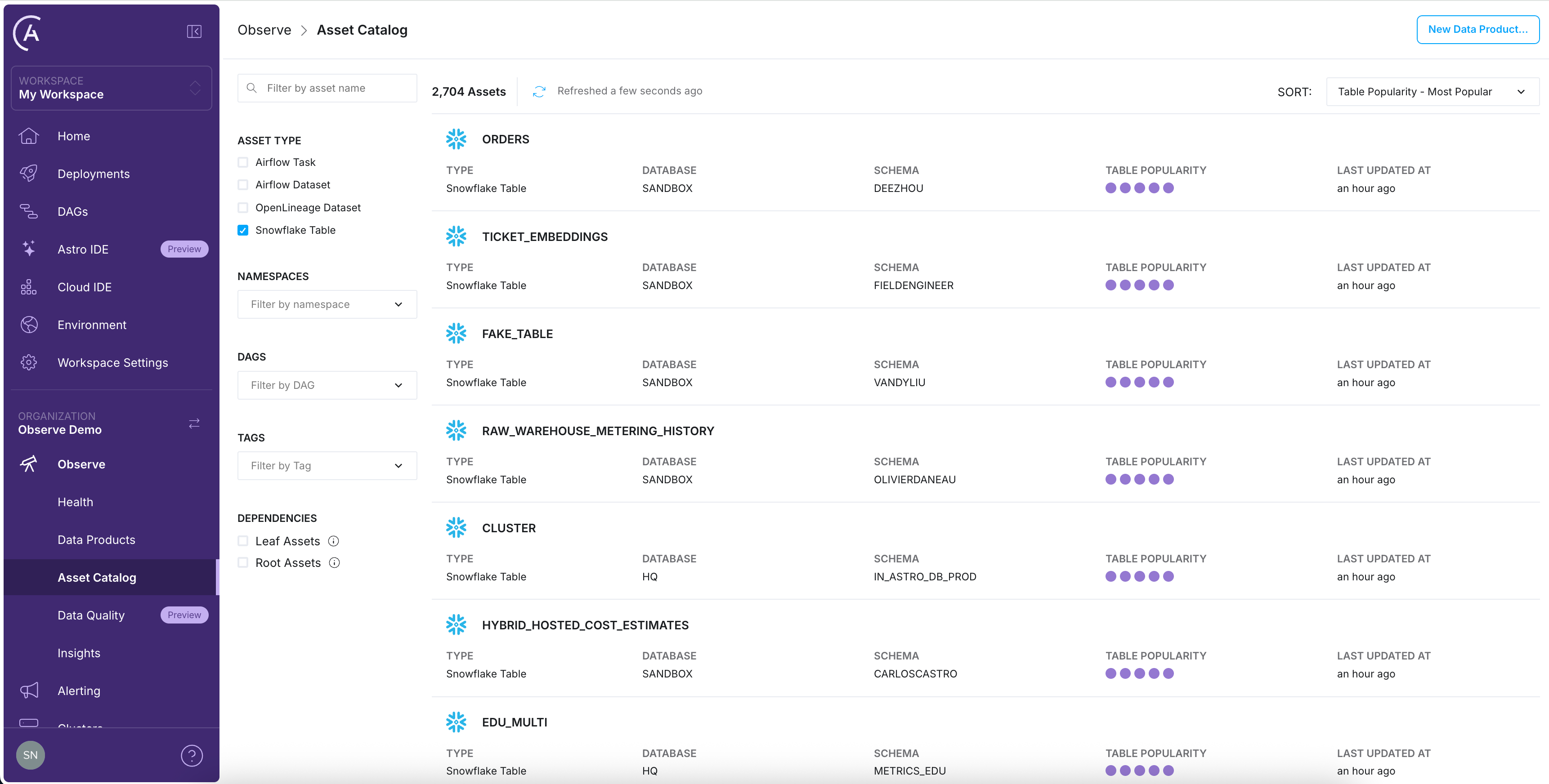
- End-to-End Lineage: View table-level lineage in context of your pipelines to trace issues from root cause to business impact (coming soon).
- Track key tables within Data Products: Add key business tables to a data product and instantly view all upstream assets that contribute to it, including pipelines and tasks, without manual work (coming soon).
- Data Quality Dashboard: Get a real-time snapshot of overall data quality health, active monitors, and threshold violations—all in one place.
By combining orchestration and observability, Astro Observe links data quality directly to pipeline execution health, making it easy to trace issues back to specific Airflow tasks or code. This unified approach enables faster root cause analysis, proactive remediation, and greater trust in the data powering analytics and AI initiatives.
From Siloed Monitoring to Unified DataOps
Data quality issues don’t exist in a vacuum, they’re symptoms of broken processes across complex pipelines. That’s why observability without orchestration context falls short. By uniting observability with orchestration, organizations gain end-to-end visibility and actionable insight into where, how, and why data issues occur – so teams can resolve them faster and prevent them entirely.
The combination of orchestration, data observability and data quality monitoring creates the foundation for a true DataOps platform, where reliability is built into the system rather than bolted on afterward.
Join the Waitlist
We’re currently offering preview access to a limited group of design partners as we refine the experience. If you’re interested in exploring Astro Observe’s data quality functionality, join the waitlist to be notified as the private preview expands.
This release is one of the many powerful enhancements to come in Astro Observe designed to help data teams gain full visibility into their stack and build more reliable, trustworthy data products.
If you’re currently evaluating observability solutions and want to see how orchestration-aware monitoring can elevate your data observability strategy, book a demo to explore Astro Observe today.